New camera design can ID threats faster, using less memory
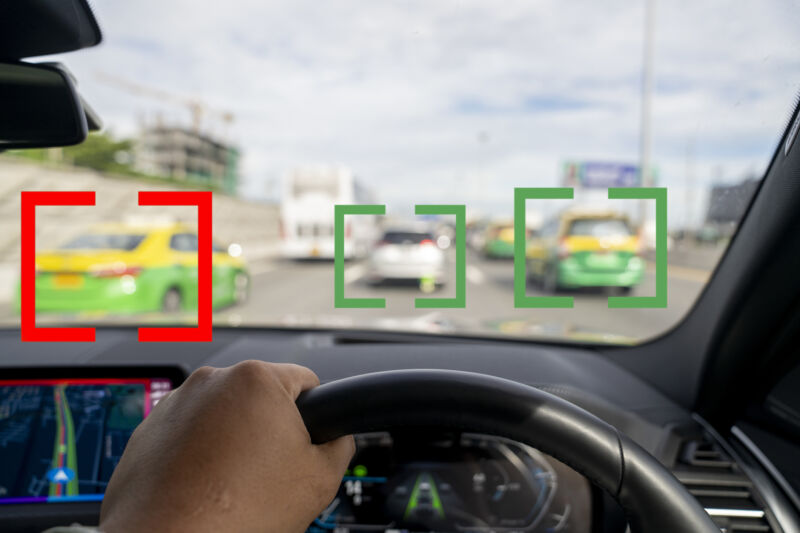
Enlarge (credit: Witthaya Prasongsin)
Elon Musk, back in October 2021, tweeted that “humans drive with eyes and biological neural nets, so cameras and silicon neural nets are only way to achieve generalized solution to self-driving.” The problem with his logic has been that human eyes are way better than RGB cameras at detecting fast-moving objects and estimating distances. Our brains have also surpassed all artificial neural nets by a wide margin at general processing of visual inputs.
To bridge this gap, a team of scientists at the University of Zurich developed a new automotive object-detection system that brings digital camera performance that’s much closer to human eyes. “Unofficial sources say Tesla uses multiple Sony IMX490 cameras with 5.4-megapixel resolution that [capture] up to 45 frames per second, which translates to perceptual latency of 22 milliseconds. Comparing [these] cameras alone to our solution, we already see a 100-fold reduction in perceptual latency,” says Daniel Gehrig, a researcher at the University of Zurich and lead author of the study.
Replicating human vision
When a pedestrian suddenly jumps in front of your car, multiple things have to happen before a driver-assistance system initiates emergency braking. First, the pedestrian must be captured in images taken by a camera. The time this takes is called perceptual latency—it’s a delay between the existence of a visual stimuli and its appearance in the readout from a sensor. Then, the readout needs to get to a processing unit, which adds a network latency of around 4 milliseconds.